Conversational AI – the Good, the Bad and the Promising
Think of this as your AI roadmap – from the emergence of advanced Generative AI to delving into the complexities of multi-modal interactions and grasping the significance of the revolution in vector databases like Milvus or Pinecone.
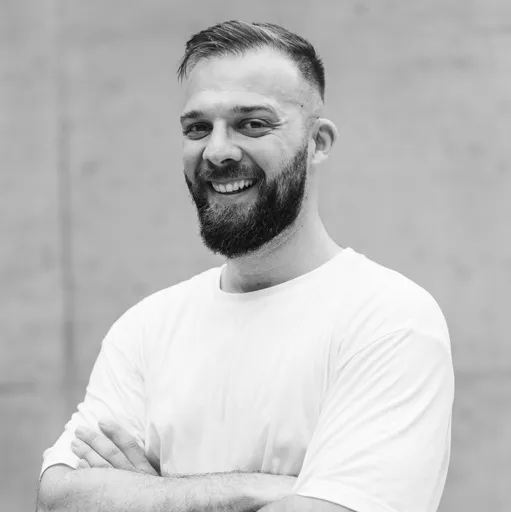
Let’s uncover the trends and practical aspects that showcase Conversational AI as more than a buzzword – it’s a language that is actively shaping the future.
Navigating the Evolution of Conversational AI: GenAI Takes the Lead
The domain of artificial intelligence is undergoing a transformative era, especially within Conversational AI, where one of the most notable trends is the shift towards Generative AI (GenAI). These advanced agents, armed with cutting-edge algorithms, are ushering us beyond the realm of traditional chatbots.
As an example, take a closer look at LAQO, the pioneer of 100% digital vehicle insurance in Croatia. Their cutting-edge digital assistant, affectionately named ‘Pavle,’ not only showcases but practically flaunts the immense potential of GenAI.
Pavle adeptly maneuvers through complex customer queries, unveiling a level of precision that sets a remarkable standard in the Conversational AI landscape. Impressively, he resolves 30% of customer queries.
Embarking on the Era of Multi-Modal Interaction
Another significant trend is the integration of multi-modal inputs and outputs in conversational platforms. Venturing beyond mere text, these systems seamlessly embrace voice, image, and video inputs, akin to a communication mirroring human interaction.
Yet, the true magic lies in the challenge at handcrafting algorithms with the finesse to precisely process and respond to this mix of inputs, crafting an experience so seamless that it effortlessly adapts across diverse modes, creating a rich and engaging experience for users.
What Lies Beyond? Exploring Current Frontiers and Challenges in Research
And what is the focus of the current research in Conversational AI? Understanding user behavior and designing task-oriented agents that can maintain trust and engagement.
A significant puzzle in our landscape is how to consistently keep users engaged. Agents need to handle diverse inputs creatively, often by rephrasing user queries and providing varied responses to enhance the interaction. It’s a practical aspect of our work that contributes to enhancing user interactions.
Additionally, the development of multi-modal Large Language Models (LLMs) such as CLIP (encoding images and video frames) and Whisper (encoding audio data) is a key research area. These models are essential for encoding and interpreting varied data forms, pushing the boundaries of conversational agents’ capabilities. However, they also bring challenges in ensuring accurate and contextually relevant responses.
Conversational Recommender Systems as a New Layer of Interaction
Conversational recommender systems are on the forefront of innovation, evolving beyond mere suggestion mechanisms. These systems now focus on the timing and manner of recommendations, adding a layer of complexity to AI-user interactions.
But, the complexity of Conversational Recommender Systems lies in striking a delicate balance in when and what to recommend. What does it mean? It performs well in rating prediction, but poorly in sequential and direct recommendation tasks.
It also has a high potential in generating explanations and summaries, but the challenge still lies in generating contextually appropriate recommendations, coupled with explanations and summaries that resonate with users.
Tackling AI ‘Hallucinations’ and Inappropriate Responses
One significant challenge in Conversational AI is addressing the issue of ‘AI hallucinations’, where models generate off-topic or irrelevant responses.
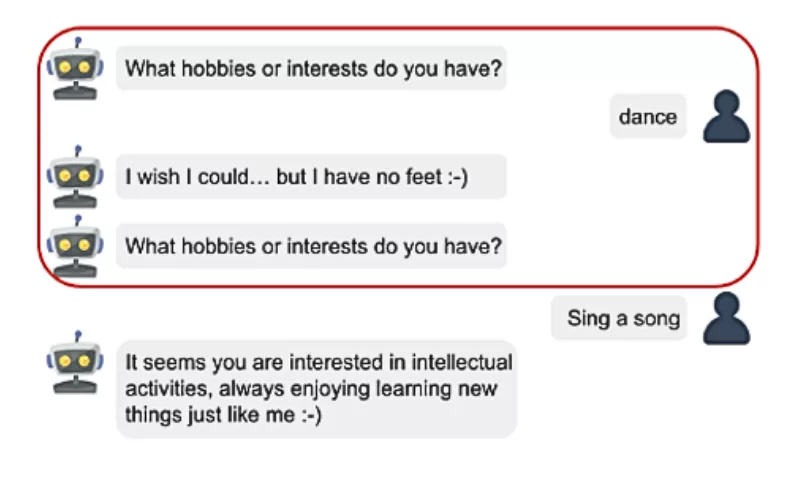
Addressing this type of challenges involve starting a new conversation to reset context (let’s call this session management) and continuous evaluation using metrics like cosine similarity, BLEU/ROUGE, or BERT scores.
Keeping AI interactions relevant and appropriate is key, and it’s an ongoing process – we adapt continuously based on user feedback and analyze responses to ensure a seamless experience.
The concept of using Large Language Models (LLMs) like GPT-4 as human judges is one recent trend to tackle the problem of hallucinations. The hypothesis here is that (some) LLMs have implicitly captured some notion of dialog quality and can therefore be used for evaluating a conversation. As such, creating open judgment-specific LLMs that can match GPT-4 evaluation skills is also one of the new frontiers from research communities that focus on Conversational AI.
What’s next?
Summing it up, the landscape of Conversational AI is buzzing with exciting developments like GenAI and multi-modal platforms. While these innovations bring promises of better user experiences, they also come with challenges.
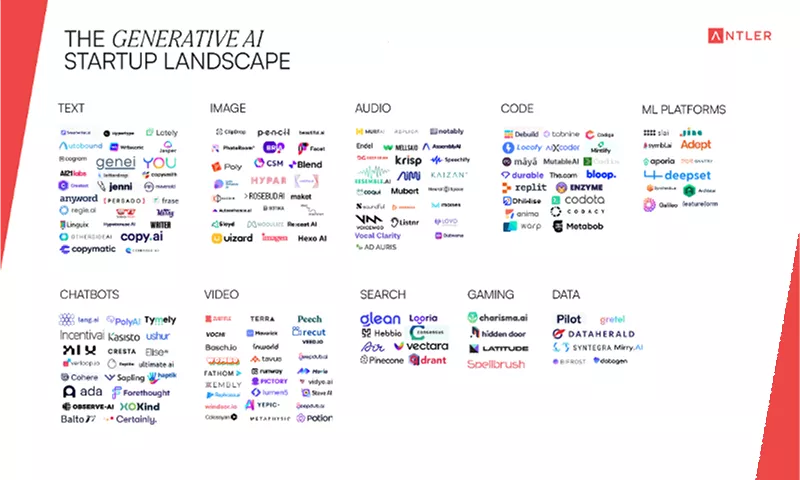
The path ahead involves refining response accuracy, enhancing user engagement, and delivering content just right. It’s a journey towards more interactive, user-friendly systems, a constant adaptation to the dance of human-AI interaction complexities. Cheers to the exciting road ahead!