How to become a data-driven organization
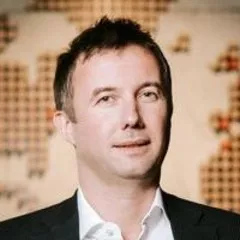
What does it mean to be data-driven?
Being data-driven means a business makes all its strategic and everyday operational decisions based on data analysis and interpretation. On a practical level, it requires forming a hypothesis, continually listening to the evidence, and asking additional questions. Sometimes, the answers to those questions will confirm the hypotheses, but in other cases, there may be a surprise. Therin lies the opportunity for innovation.
With advances in the way data is stored and organized, increases in computing power, and new analytics techniques, the velocity of this cycle has increased tremendously. From formulating the hypothesis, to the questions and data investigation, through to the decision phase can now be completed in a relatively short period, provided a good strategy is in place.
For businesses looking to optimize their marketing spend, being guided by data allows them to effectively drive customer engagement across channels, make accurate predictions about what customers want and need, and ultimately deliver the right piece of content at the right time to secure sales. This has become even more important with Google currently phasing out third-party cookies from Chrome browsers. Being able to effectively utilize first party data is now imperative.
Today we can do things with data that we could not have imagined in the past – from the real-time diagnosis of diseases based on data patterns to the automation of decision making in the marketing funnel. However, businesses can only capitalize if they organize their data and analytics to increase velocity and improve the quality of outcomes.
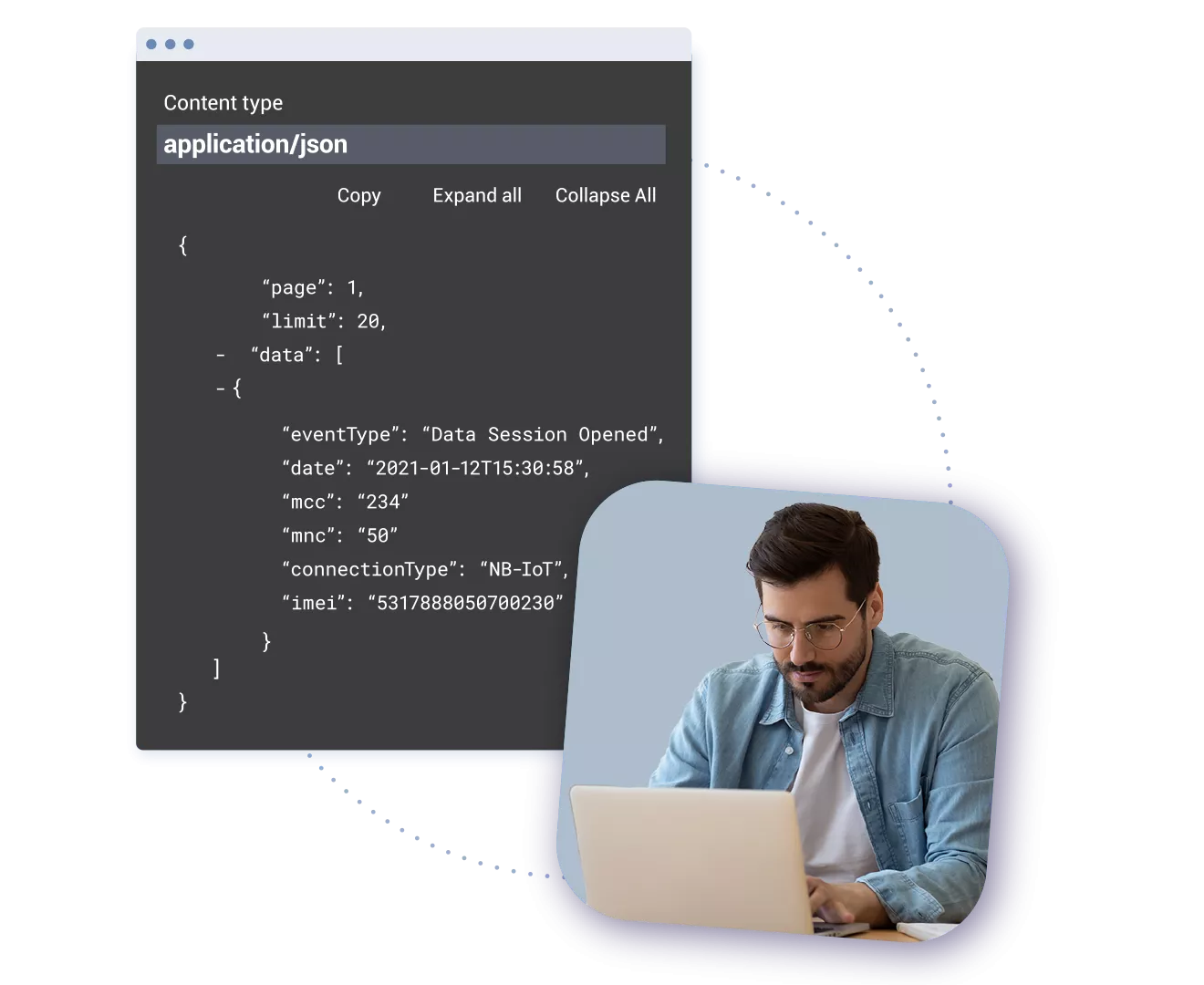
The data transformation challenge
There are three primary challenges for companies looking to be more data driven: strategy, analytics methods, and successful adoption within the company.
Most data transformations fail because of the ‘human’ factor. Staff don’t understand their own role in the transformation and the benefits it will bring, and the process stalls without a common sense of purpose.
To prevent this, project leaders need to first build a solid analytics strategy and get the commitment of management to scale that strategy within the organization. They then need to develop a clear data plan, including data ontology, a master data model, governance on who can write and access the data, but also when imperfect data is ok, and having a suitable tech stack to extract, move and use data effectively.
Finally, companies need to focus on the ‘last mile’ by embedding data outputs in core processes in order to drive change management and full adoption by internal teams.
The five must-haves to become a data-driven company
1. Strategy
To start it’s essential to establish a clear strategy that articulates what an organization wants to achieve with analytics. Transformations often fail because companies try to extrapolate pilot projects across the whole organization.
Organizations that do it successfully have a clear plan for transforming their entire business domain using data and analytics. Instead of a one size fits all approach, they should launch a series of use cases that drive end-to-end transformation across the business.
2. Investment
Successful companies spend more than other organizations on analytics. Profitable scalers spend more than 25% of their IT budgets on analytics, including expenditures such as data, technology, analytics talent, and embedding analytics into business-process workflows.
This investment is due to further increase as 75% of leading companies are reporting plans to boost their analytics spending. Most importantly, a lot of companies allocate much of this budget toward the biggest challenge companies face — extracting value from analytics — the so-called ‘last mile’ which involves embedding analytics into the core of all workflows and decision-making processes.
3. Quality of data
There is a famous saying “garbage in, garbage out” when it comes to data. It is important to have a clear data strategy that includes a data catalogue, a master model, ways to move and extract data, and ways to check data integrity and quality.
Successful companies have built these systems end-to-end from collecting data, to the data that’s fed into their models. They also have good systems for assessing when data is “good enough” versus when it has to be perfect. This is often an impediment to adoption as time and resources are wasted seeking perfection in cases where it is not the main priority for achieving success.
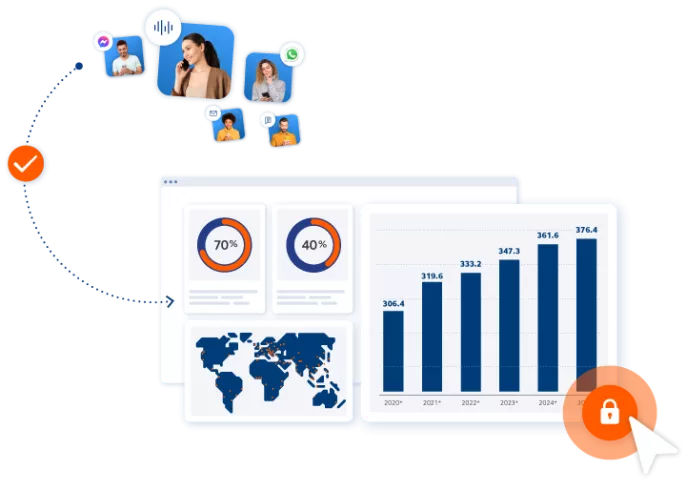
4. Developing analytics models and methods
Successful companies have a clear methodology for developing analytics models, interpreting insights, and deploying the new capabilities that they build. Leading analytics companies not only focus on model development through their methodologies, but also work to continuously maintain and upgrade models as part of a sophisticated model-management function.
Many analytics leaders constantly test and upgrade the quality and performance of analytics models using a challenge and test approach that compares existing data sources and algorithms against new and potentially better alternatives. Leading companies are also more likely to use sophisticated analytics techniques, such as reinforcement learning and deep learning, which can provide a substantial lift in value over more traditional analytics approaches.
5. Source and nurture analytics talent
Attracting and retaining talent and establishing efficient ways of working are key to data transformation projects. Successful companies have many deep functional experts in different aspects of data science and deploy around 25 to 50 full-time employees per 1,000 in data and analytics roles.
In addition, there needs to be a full-blown talent strategy on how to develop, retain and grow analytics staff with a clearly defined training and project curriculum, as well as career paths and personal development opportunities (including R&D and cutting-edge projects).
In addition, these companies need to organize analytics teams in very agile ways, with strong cross-functional teams mixing business, analytics and analytics translators operating at a high pace in a test-and-learn mode.
Transforming a work culture to be data-driven
The main obstacle for successful adoption of a data-driven approach is not in the technical issues as much as in the culture shift required.
It is a critical topic to address. In a nutshell, organizations have to build capabilities and ways of working that drive the levels of data literacy and usage required for success. They also need to develop trust in the models used in key decision-making processes.
Everything else flows from these goals, but there are proven change tactics that can help. These include:
- Signaling the importance of data and analytics by top management
- Steering behaviors by using the right metrics for analytics programs
- Investing in capability building e.g. the right training for all employees to increase data literacy
- Treating analytics as a product with the goal of supporting employees with data and analytics
- Standardizing ways of working and integrating data-scientists into the organization
- Communicating analytics program successes, explaining analytics choices, and being transparent about the uncertainty and limitations of models
Future trends for the data approach
We foresee three major trends developing in the next 5 years.
- The first is around building data ecosystems that transcend individual businesses, where we will be able to interoperably connect data from different sources for given use cases, deal with missing or anonymized data, and support any model creation with this. It follows that consumers will become more willing to provide consent to use their data if they see tangible rewards for doing so.
- The increased automation of data processing, for example analytics systems that are able to do data cleansing and transformation in a semi or fully automated way. This is important because today 60 to 80% of time in analytics projects is spent on preparing data and dealing with missing data. With these changes companies will be able to increase output from their analytics teams.
- The third trend will be in scaling analytics to become much more like scaling software with various data and analytics assets coded as libraries, and being able to be shared and used across the organization in a modular way.
How data can enrich the customer experience
At Infobip we are on a mission to leverage data to recreate a full end-to-end customer journey in any channel that the customer prefers. Of course, people don’t use just a single channel, so we also need to be able to create “memory” across the different channels a customer uses to communicate with a business.
For instance, imagine if you could buy the clothes you like from your favorite chat app (e.g., WhatsApp) and that you can accomplish all tasks from the initial search, through to purchase and customer support without leaving that chat app.
Now imagine if one day you decide to call the store, and once you connect, they know all your history and all your preferences, so you don’t need to educate that business again and again on your previous interactions, with everything automatically personalized just for you.
This would have a huge impact on engagement, on experience, and would save people time – the most important commodity in hectic modern lives.
The same principle can be applied beyond commercial use cases where it could have a fundamental impact on people’s lives. Imagine healthcare – a frictionless patient journey across multiple channels could dramatically speed up the process from booking an appointment, to diagnosis, treatment, and aftercare. It could literally be a lifesaver, but it can only be achieved if all organizations involved transform to be data-driven.
Set yourself up for exponential growth
Learn from our leaders and experts the latest trends that will set you on the path of success.
Read more